Rogier Hintzen (1), Jake Lever (2), Dan Sosa (3), Julien Fauqueur (1), Roxana Lupu (1), Mark Davies (1) and Russ B Altman (4)
1: BenevolentAI, 2: Department of Biomedical Data Science, Stanford University, 3: School of Computing Science, University of Glasgow, 4: Departments of Bioengineering and Genetics, Stanford University
BenevolentAI and the Helix Group at Stanford University — led by Professor Russ Altman — share insights into their work on developing more effective methods to extract knowledge from biological and clinical information.
Summary / takeaway points:
- The AI research partnership looks at the role of context in information extraction, with an emphasis on more granular, precise and high-resolution representations of biomedical information
- The researchers have observed the importance of modeling biological context to refine/extract more nuanced and accurate PPI networks/interactions
- Moving forward, the two teams will expand the scope of the collaboration to include document-level features and create context-specific networks
Intro to the collaboration:
Both BenevolentAI and Stanford have a strong track record in using AI to tackle some of the biggest challenges in biology and medicine. Through this partnership, researchers from the two organisations have pooled their expertise in AI, natural language processing and creating knowledge graphs to reason about drug discovery, with the aim of finding more effective methods to extract knowledge from biological and clinical information. The research focuses on the role of context in information extraction, and aims to represent information more precisely in order to enhance the downstream target and drug predictions made by machine learning models.
Intro to the project:
Scientific researchers are generating more data than ever before and there is a great potential to use AI models to extract information from this data to predict novel drug targets or molecules. While this potential has already been realised by some, several challenges remain in unlocking the full potential of AI in this area of drug R&D. Principally, these models require databases of information which compile the relationship between biomedical entities like genes, chemicals and diseases, often structured as a graph. Relying on manually curated databases to construct these graphs results in sparse coverage of biological relationships. There is a well-recognised opportunity to extract additional relationships from the scientific literature using AI. To do so, however, presents a challenge due to the fact that the characteristics of these relationships, such as whether the interaction is positive or negative, can often appear contradictory in different scientific manuscripts.
These contradictory characteristics may be because the scientific results truly are contradictory, but it is also likely that relationships are contingent on the biological context in which they occur, depending on factors like: the location - such as tissue or cell type - in which the two entities are interacting (Nam et al., 2018 ); the state of that location (Dai et al., 2018 ); or whether the relationship was described in an organism in a healthy or diseased state (Kuzmanov & Emili, 2013 ). Two proteins may have a strong interaction in one cell- or tissue-type and may not have that interaction in another, so it becomes critical to associate extracted facts with the details of their context, in order to build accurate models. See an example in figure 1.
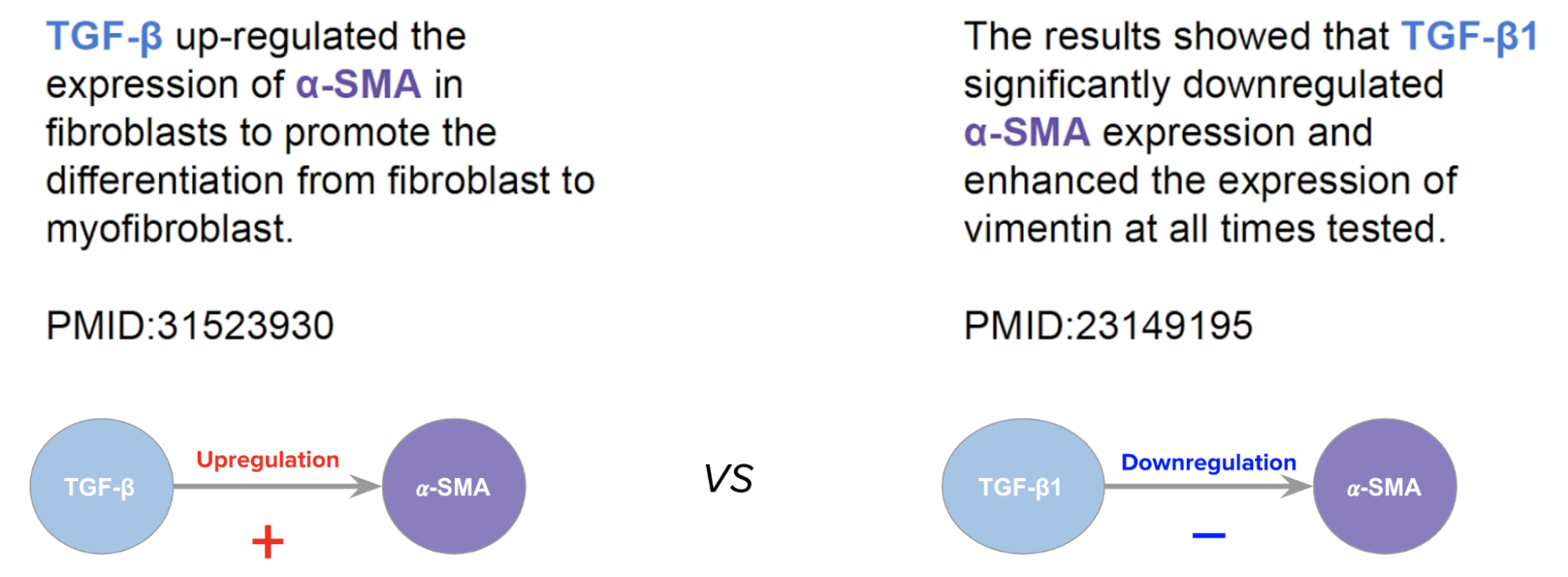
Figure 1: Example of a contradictory interaction between the same pair of genes in two documents.
In this exciting collaboration between BenevolentAI and Stanford University, we address these two challenges: we have developed methods to extract signed, causal gene-gene relationships from text and then associate these relationships with a specific biological context to reconcile apparently contradictory facts.
First challenge: extracting signed, causal gene-gene relationships from text
For the first challenge, we focussed on extracting gene-gene regulatory events, where one gene is described as controlling the expression of another with a specific biological direction (the “sign” of the relation). For example, a sentence relating the downregulation of one gene by another might read:
leptin inhibited the expression of MMP-1 in LX-2 hepatic stellate cells;
whereas another sentence relating the upregulation for the same pair of genes may read:
human chondrocytes stimulated with leptin upregulate MMP-1.
Relationships with such a strong representation of ‘sign’ (negative in the first example and positive in the second) ensure we extract relationships for pairs of genes with examples of apparently contradictory positive and negative interactions.
Using dependency parsing and a novel tree traversal method, varied textual mentions involving two gene entities were mapped to relatively few dependency paths (see figure 2).
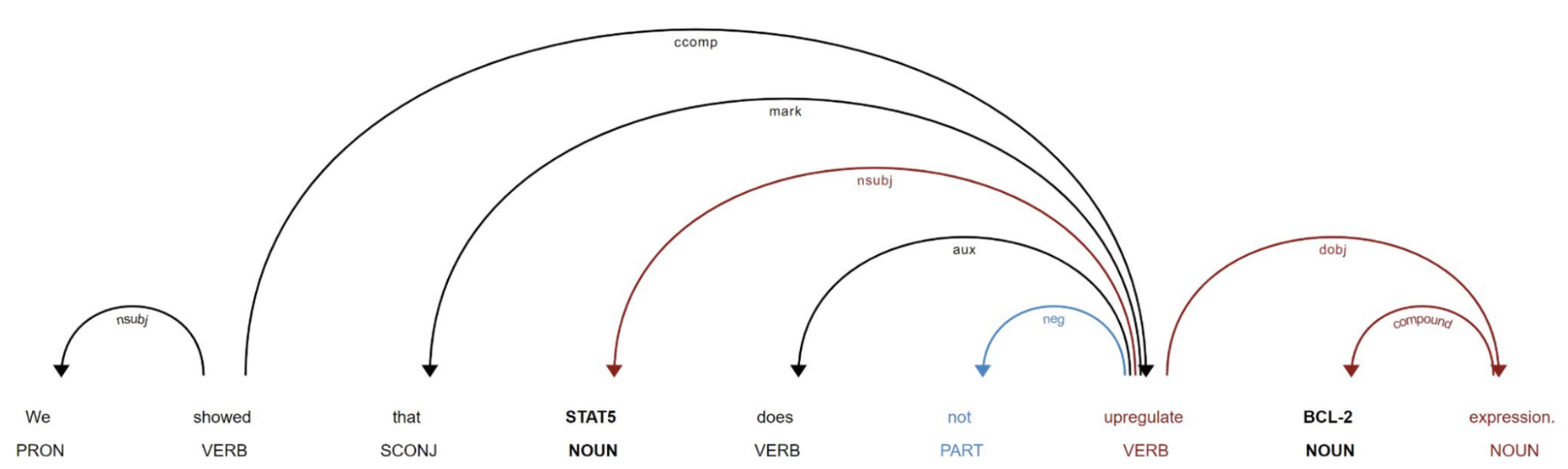
Figure 2: The augmented dependency path (visualised with displaCy)
The traversal algorithm extends previous work by BenevolentAI and Stanford University by incorporating important information relevant to the sign of the relationship, that lies off the direct dependency path between the two gene entities. Experts from Stanford then annotated the most popular dependency paths. This had an important multiplier effect, as each annotation translated into the annotation of multiple sentences corresponding to this dependency path.
Second challenge: determining the biological context of an extracted relationship
The second challenge focussed on establishing a relevant context specific to a span of text describing a relationship. Pairs of sentences that described an apparently contradictory regulatory relationship were shown to expert annotators, who were asked to annotate whether the sentences truly described contradictions in the literature, or whether contextualising information would mitigate the contradiction. For example, the two sentences above indicate that the two ‘contradictory’ relationships occur in very different cell types (hepatic stellate cells versus chondrocytes). This annotation exercise provided a high-quality set of context annotations for gene-gene regulatory interactions reported in the literature.
Stanford University researchers then implemented and expanded on an existing model for predicting the context of a relationship extracted from text (Noriega-Atala et al., 2019). This model uses structural features of the text, like the distance between sentences expressing relationships and text spans that might represent an appropriate biological context, to make relationship-context predictions.
The researchers extended this model by adding in additional features describing where in the document context and relationship text spans were found, as a heuristic for determining their relevance to one another. For example, a reader might expect that if both the relationship and context spans are extracted from the discussion section, the context may be more likely to qualify the relationship. Other paper-level features were incorporated including whether or not the context term occurs in the publication's MeSH headings (a set of concepts relevant to the main findings of the paper as annotated by manual curators).
The annotated context in the First Challenge was used to assess the resulting model, alongside the publicly available set of annotations in Noriega-Atala et al., 2019.
Early results
Annotations
The exercise of extracting apparently contradictory gene-gene regulatory events and the annotation of relevant context were invaluable in understanding the problem.
The first important discovery was that document-level context was often insufficient to describe the context of a gene-gene regulatory relationship, as different contexts are often discussed at a more local scale in a manuscript.
The expert annotations showed that by far the most relevant piece of contextual information was the cell type in which the regulatory relationship was observed, representing the majority of the annotations (see figure 3). Rarely ever were statements thought to be irreconcilable, once contextualising information - like cell type or experimental treatment - were considered.
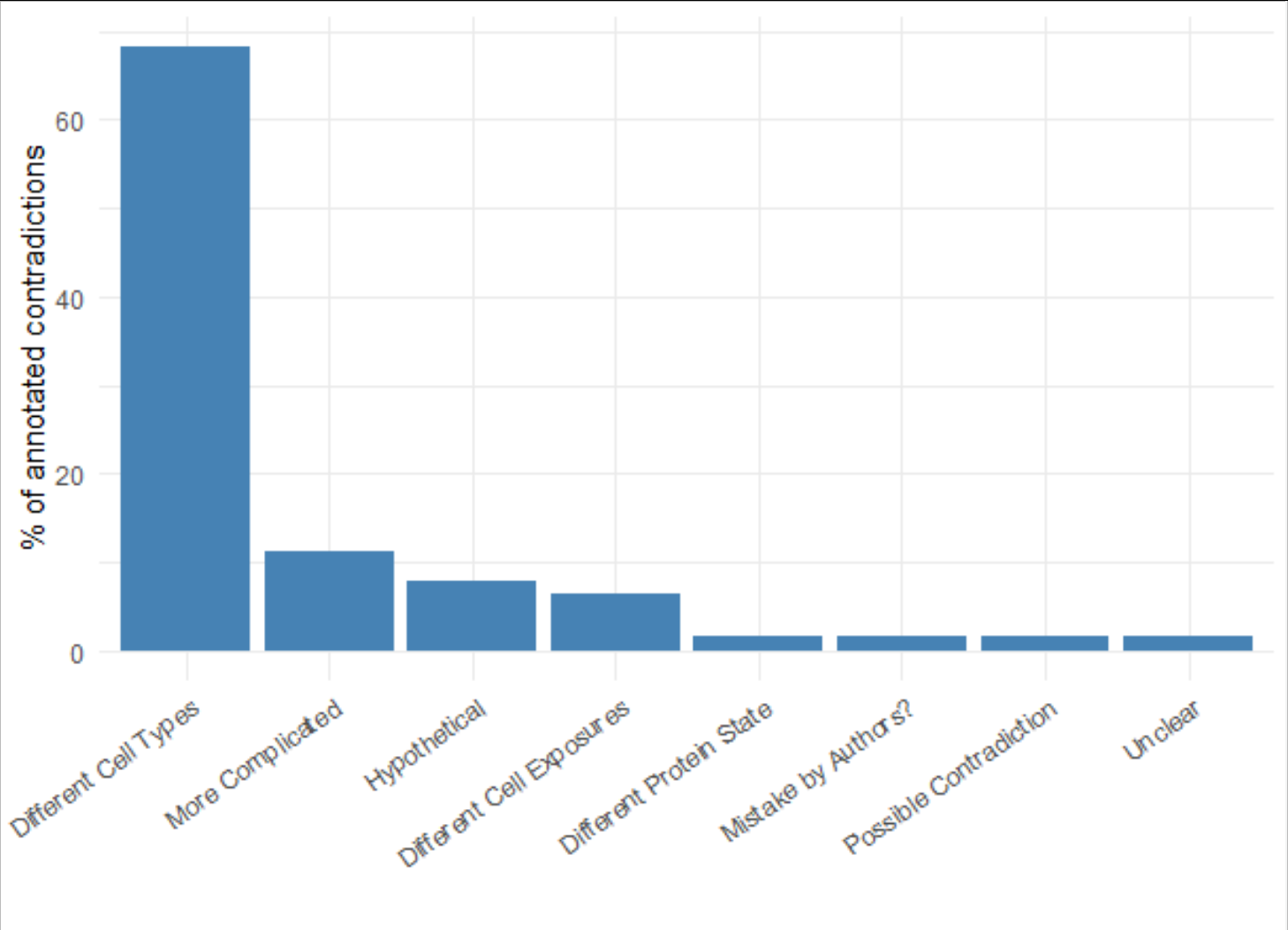
Figure 3: The main cause of contradiction between 2 statements was found to be “Different Cell Types”, i.e. different cell-lines, tissue types or diseases
Finally, researchers found that in a substantial number of cases the relevant context could not be found in the manuscript the relationship was extracted from, and it was necessary to follow references to identify the appropriate context. These observations support the idea that all biological texts should clearly specify at least the most basic contexts for their observations including organism, cell-type and perhaps subcellular localization.
Predicting relation context
The modelling exercise showed that new section-level features were highly important to the model predictions. While the new features did not boost performance against the published model, an error analysis showed encouraging trends. The model was provided with negative examples by sampling from all un-annotated relation-context pairs. However, many of these were either missed by human annotators, or should not be considered hard negatives, as the predicted context was a closely related concept to the annotated context. For example, in one instance the new model using structural document features predicted ‘vesicles’ to be the context for a relation, whereas the annotators of the published corpus had annotated ‘Golgi’. In other cases, the published annotations of relation-context pairs were shown to be over-precise and, during the model error analysis, were not supported by a review of the annotations by this set of researchers.
Moving forward
The first stage of the collaboration has helped Stanford and Benevolent researchers gain an understanding of the types of context that are important to qualify biological relationships and has given them an opportunity to build a set of competitive baseline models.
The team are planning exciting routes forward to develop a state of the art context extraction model. We will use this to create context-specific regulatory networks across the entire PubMed biomedical manuscript collection which will add more confidence and accuracy to machine learning models used downstream in the drug discovery process. We ultimately hope this research will extend the potential of artificial intelligence in drug discovery, and help scientists discover and develop better medicines.
About The Helix Group
The Helix Group at Stanford University is directed by Prof. Russ Altman. It is part of the Bioengineering and Genetics departments and focuses on computational informatics, data science and AI technologies for molecular medicine, with a focus on drugs, drug response and drug repurposing.
About BenevolentAI
BenevolentAI is a leading, clinical-stage AI drug discovery company. Through the combined capabilities of its AI platform, scientific expertise and wet-lab facilities, BenevolentAI is well-positioned to deliver novel drug candidates with a higher probability of clinical success than those developed using traditional methods. BenevolentAI has a consistently proven track-record of scientifically validated discoveries. The BenevolentAI Platform™ powers a growing in-house pipeline of over 20 drug programmes, spanning from target discovery to clinical studies, and it maintains successful commercial collaborations with leading pharmaceutical companies. BenevolentAI also identified Eli Lilly's baricitinib as a repurposing drug candidate for COVID-19, which has been authorised for emergency use by the FDA. BenevolentAI is headquartered in London, with a research facility in Cambridge (UK) and a further office in New York.
Back to blog post and videos