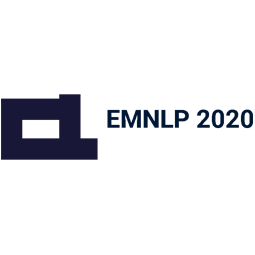
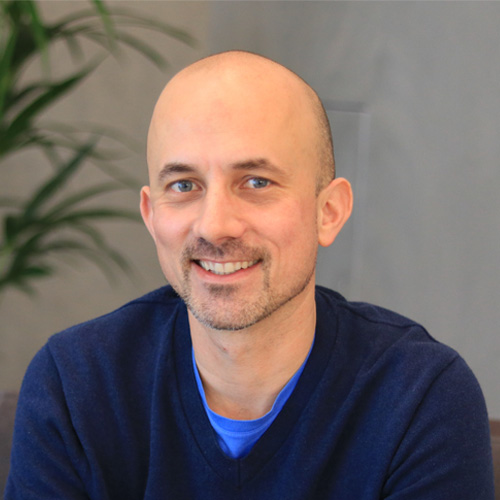
LOUHI 2020: The 11th International Workshop on Health Text Mining and Information Analysis.
20 November | 09:30 | Register here
Simple Hierarchical Multi-Task Neural End-To-End Entity Linking for Biomedical Text
Recognising and linking entities is a crucial first step to many tasks in biomedical text analysis, such as relation extraction and target identification. Traditionally, biomedical entity linking methods rely heavily on heuristic rules and predefined, often domain-specific features. The features try to capture the properties of entities and complex multi-step architectures to detect, and subsequently link entity mentions. We propose a significant simplification to the biomedical entity linking setup that does not rely on any heuristic methods. The system performs all the steps of the entity linking task jointly in either single or two stages. We explore the use of hierarchical multi-task learning, using mention recognition and entity typing tasks as auxiliary tasks. We show that hierarchical multi-task models consistently outperform single-task models when trained tasks are homogeneous. We evaluate the performance of our models on the biomedical entity linking benchmarks using MedMentions and BC5CDR datasets. We achieve state-of-theart results on the challenging MedMentions dataset, and comparable results on BC5CDR.
SustaiNLP 2020
20 November | Time TBC | Register here
Learning Informative Representations of Biomedical Relations with Latent Variable Models
Extracting biomedical relations from large cor-pora of scientific documents is a challengingnatural language processing task. Existing ap-proaches usually focus on identifying a rela-tion either in a single sentence (mention-level)or across an entire corpus (pair-level). In bothcases, recent methods have achieved strong re-sults by learning a point estimate to representthe relation; this is then used as the input toa relation classifier. However, the relation ex-pressed in text between a pair of biomedicalentities is often more complex than can be cap-tured by a point estimate. To address this is-sue, we propose a latent variable model withan arbitrarily flexible distribution to representthe relation between an entity pair. Addition-ally, our model provides a unified architecturefor both mention-level and pair-level relationextraction. We demonstrate that our modelachieves results competitive with strong base-lines for both tasks while having fewer param-eters and being significantly faster to train. Wemake our code publicly available.
You can find the paper published here, and the code can be accessed on GitHub here.
The lead author of this paper was Harshil Shah, our Machine Learning Intern.
Speakers
Maciej Wiatrak
Machine Learning Engineer
Maciej is a Machine Learning Engineer at BenevolentAI, where he focuses on Natural Language Processing (NLP) and its applications in drug discovery. In the past he has worked in research labs at University College London and University of Edinburgh on topics such as multi-agent reinforcement learning and generative adversarial networks. Recently, he has become interested in geometric deep learning and utilising different manifolds for effective representation learning.
Juha Iso-Sipila
Senior Lead AI Scientist
Juha has MSc (1996) and PhD (2008) degrees in Computer Science from Tampere University of Technology. After graduation he worked for Nokia in Finland and in China, developing speech recognition, text-to-speech synthesis and predictive text input technologies for Nokia. In 2013 Juha relocated to London to work as Head of Research at SwiftKey. During this time SwiftKey launched a neural network based mobile predictive text input system. Juha has been at BenevolentAI from 2017. His main interests are in Deep Learning for biomedical NLP and distributed computing using Apache Spark.
Julien Fauqueur
Lead AI Scientist
Julien Fauqueur is a Lead AI Scientist at BenevolentAI. He has developed natural language processing methods for biomedical information extraction and, since this year, he has been the AI Lead for the Chemistry group. He holds a PhD in computer science from INRIA in France. While a research associate at the University of Cambridge, then as a senior researcher in industry, he developed new methods and products using computer vision and machine learning. He co-authored around 30 academic publications and 5 patents.
Back to events