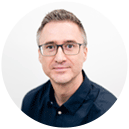
Mark Davies, our SVP Informatics and Data, discusses how BenevolentAI is innovating to fully leverage biomedical data in drug discovery and to ensure that the integrated view of data we create is more impactful than the sum of the individual data sets.
Constructing a strong data foundation
Part of my role at BenevolentAI is to ensure that we make the best use of an ever-expanding and complex biomedical data landscape to accelerate drug discovery. We purposefully leverage a wide range of data types from over 85 data sources to achieve the most comprehensive representation of biomedical knowledge possible. Unlike traditional drug discovery, this enhances our ability to formulate novel hypotheses and work in any disease area or indication, as it removes the data and therapeutic area silos.
Our approach is underpinned by data that we extract and process from the scientific literature, internal and external experimental data, and external structured data resources, such as network biology data sets, pharmacological data, chemical structures, various types of omics data, genetics data, clinical trial data and much more. We have not simply standardised and combined available data sets, but have developed machine learning models that extract relationships between different types of data, enabling our drug discoverers to see the bigger picture and make new connections.
The output of all of these efforts is a vast and powerful Knowledge Graph that represents not only what is known, but more importantly, what should be known about a disease. This graph places more than 350 million biomedically relevant relationships in the hands of its users and forms the basis for our drug discovery programmes. The graph achieves this by powering AI tools that enable scientists to derive novel insights and build hypotheses regarding mechanisms driving different diseases to ultimately discover new and better treatments.
However, the process of building the Knowledge Graph’s data foundation is incredibly challenging, and requires us to extract relationships from data sets that often use inconsistent nomenclature, include different properties, and may contain gaps or otherwise be incomplete. Here's a brief summary of how our teams meet some of these challenges.
Extracting new relationships from limited data
Machine learning models require databases of information that include relationships between biomedical entities like genes, chemicals and diseases. However, relying only on manually curated and currently available databases would lead to sparse coverage of biological relationships; as such we have developed ways to extract additional relationships from different data sources to add to our Knowledge Graph and use in our models.
Identifying the best data to use in machine learning models is a multi-parameter problem, as it requires a good understanding of the strengths, weaknesses and gaps of multiple sources. We must consider, for example, if one key parameter is missing from a given data set that is otherwise high quality, can we find another data source that provides this parameter and then work with these two complementary data sets to ensure that, when combined, they create an integrated view. But despite our best efforts, we may still end up with gaps across our sources, and in addition we sometimes have to work with small data sets or data that is unlabelled. For this reason, we need to understand what we can extract from the limited data that we do have. To address this problem, we established a team whose remit is focused exclusively on learning from limited data, with recently published work focused on ways to [integrate many small and heterogeneous omics datasets https://www.benevolent.com/research/contrastive-mixture-of-posteriors-for-counterfactual-inference-data-integration-and-fairness], which helps increase availability of disease-relevant omics data.
Augmenting data usability
It’s not just about having data: a lot must be done to most data in order to integrate it into our systems and make it usable to support drug discovery — data rarely ever comes in a ready to go, plug-in format.
This is one of the reasons why at BenevolentAI we are adopting FAIR data principles (Findable, Accessible, Interoperable and Reusable), and we encourage others to adopt them as well. The ‘Findable’ and ‘Accessible’ principles are closely linked, and involve the inclusion of machine-readable metadata, and ensuring data and metadata can be easily retrieved and actioned by machine learning tools. In addition, the data must be interoperable (meaning that it is formatted in such a way that facilitates integration with other data sources and across systems), and optimised for reuse. To achieve this, metadata and data should be well-described and use a common language so that they can be replicated and/or combined in different settings.
Facilitating integration: formats and standardisation
As alluded to above, biomedical data is unfortunately not consistently standardised to common formats, which is a major roadblock for interoperability and reusability of data. Even well-curated data sets often have been designed for different purposes, and thus can vary in the language they use to describe the data (ontologies) and how they are structured, which makes integration challenging. However, there are fantastic efforts underway both at BenevolentAI and elsewhere to improve data formats and standardisation and address this challenge.
For example, we need models and integration frameworks: a less glamorous side of our work, but arguably one of the most vital technologies our teams develop. It is crucial for aligning different modalities of data, such as omics, literature and pharmacology data, and our success in aligning such diverse data sets has allowed our drug discoverers to surface connections that are reinforced by multiple modalities, which can help with hypothesis generation. In order to improve efficiency and speed up the drug discovery process, our teams also automate and streamline our data ingestion processes to minimise the time it takes for a given data set to have a visible impact and improve users’ decision making.
Decoding the scientific literature
Scientific literature presents a unique challenge for usability. Although many of these challenges must be addressed by scientific publishers and the larger scientific community, our teams are developing ways to extract more relationships from literature data and then better integrate those relationships with our other data sources to create a foundation on which analysis and inference-based methods can be applied.
Our natural language processing (NLP) models involve initial entity recognition and mapping onto ontologies and the representations of human biology and disease we have built; we then extract those literature-derived relationships and connect biomedical entities together to create a view of what is happening. For example, we have [developed a simpler yet effective approach to entity linking for biomedical text that does not use heuristic features https://www.benevolent.com/research/simple-hierarchical-multi-task-neural-end-to-end-entity-linking-for-biomedical-text]. However, this does not mean that we simply pull out relationships that are stated in scientific papers — with our models we can also identify novel answers to a biomedical question based on indirect evidence in the literature.
One challenge with using NLP approaches on the scientific literature is that different papers will often present data that on the surface appears to be contradictory. For example, a given protein may be shown in one paper to upregulate a gene, but another paper reports that the same protein downregulates that gene. In many cases this is because the relationships are contingent on the biological context, such as the cell or tissue type in which they occur. Thus there is a need to extract context in our NLP models. We are working on this problem with the Helix Group, a Stanford University-based research lab led by Professor Russ Altman.
Building data-driven tools and products that empower faster drug discovery
BenevolentAI’s Knowledge Graph is constantly enriched with new data. However, providing that data in a format that is accessible and useful to our drug discoverers requires tailored tools and platforms that our teams build on top of our Knowledge Graph. These tools present the most salient data to our drug discoverers, providing them with the supporting evidence as to why a target has been suggested for a particular disease and enabling them to reliably interpret the presented models so that they can weigh all of the data and come to an informed decision about selecting a target for progression.
We have gone through several iterations of building what we consider to be the best pipeline to bring everything together — the models, the data and the different engineering components — in our platform. However, this is a continuous process. We track usage of our systems to understand how our data is having an impact for our drug discoverers, and in turn, their feedback allows us to update any previously held assumptions about data and modify our processes and improve our systems so they are more useful to them.
Conclusion
The end goal of our engagement with data at BenevolentAI is to use it in the smartest way possible to improve the drug discovery process and enable better predictions, and we must constantly innovate to address different challenges. Our models and algorithms are only as good as the data that goes into them, thus it is essential that we continually refine and improve our processes to ensure our data foundations are as strong as possible.
Find more
Mark Davies at the LSX World Congress
Hear Mark’s views on how AI, machine learning and big data are disrupting the drug discovery and development landscape for faster and more accurate lead identification.
Text & Data Mining In Drug Discovery: A Conversation With Springer Nature
Hear more from Mark about how BenevolentAI leverages scientific literature, as well as other data types, to expedite drug discovery.
Back to blog post and videos