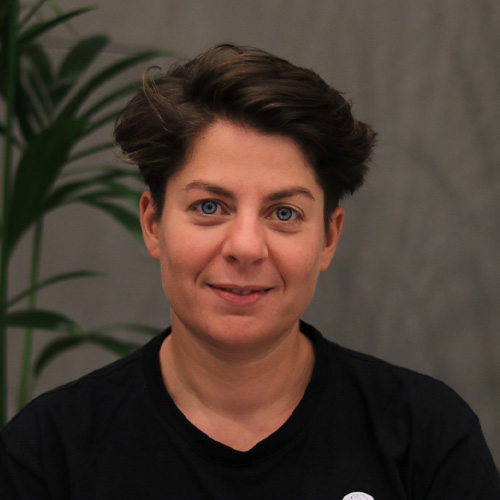
Missing information and biases in demographic information are widespread in biomedical data that underpins the entire drug discovery and development process. Machine learning solutions trained on this data need to understand and adapt for these biases to avoid perpetuating health inequities.
It is well reported that genomic research data is dominated by data from individuals of European descent even though they are far from being the majority of the global population.
In-depth analyses on the diversity of ethnicities in GWAS studies shows a vast disparity. Age, sex, ethnicity and other demographic factors can influence a patient’s outcome based on their association with long-standing healthcare and societal inequities or, although less common, by changing the efficacy of drugs based on biological differences. These imbalances contribute to existing disparities in healthcare. Thus, technologies developed with the use of such datasets may not benefit everyone.
Having shared our open-source Diversity Analysis Tool last year, we were tasked to investigate and demonstrate the lack of diversity in data as part of the State of AI Report 2021. We chose to look into the diversity of publicly available gene expression data. As a read-out of the dynamic biological state in disease-relevant tissue, this data modality is used throughout the drug discovery process, yet there has not been any known analysis of its diversity published. While our analyses found a similar overrepresentation of samples from Europeans, our research showed that omission of age, sex and ethnicity information presents a major data gap. The first step towards more inclusive health research involves measuring bias. This is especially important for machine learning (ML) applied drug discovery, as it enables creators of ML solutions trained on these datasets to better understand and adapt for these biases to avoid perpetuating health inequities. You can see our contribution to the State of AI Report on slide 53.
At the time of our analysis, there were 3,000 studies providing publicly available gene expression data for diseases (excluding cancer) from human samples and cell lines deposited in GeneExpressionOmnibus, ArrayExpress, the European Nucleotide Archive and the Sequence Read Archive, which in total comprised 177,201 individual samples. We accessed this data through Omicsoft DiseaseLand and post-processed the data to consolidate age and ethnicity information across the different studies.
Using our Diversity Analysis Tool to carry out this analysis was straightforward. It was primarily designed to inspire more sophisticated solutions from the wider research community and we had previously only used it on ad hoc datasets. Therefore, it was great to run the analysis successfully on such a large volume of data and to dig into the results all in an afternoon.
What stood out immediately was that for many samples, information on age, sex and/or ethnicity is not reported at all. While most samples had information in either sex, ethnicity or age, completeness of this information is lacking: 71,136 (40%) samples have no sex information of the donor, 125,374 (71%) samples have no information on ethnicity and 84,678 (48%) samples have no age information of the donor.
Whilst this missing information makes it difficult to draw concrete conclusions, digging into the data, we identified more biases and data gaps. Compared with all other ethnicities, African American / Afro-Caribbean samples are significantly underrepresented above age 55 (402 of 11,595 samples above age 55 with ethnicity information; p-value <0.001, 2-sample proportion test). Male samples are also underrepresented compared with female samples in African American / Afro-Caribbean (1,388 of 4,037 samples with sex and ethnicity information; p-value <0.001, 2-sample proportion test).
We found specific biases in samples from individuals aged under 5 years which include samples predominantly used in developmental biology research (5,128 samples) - there was a significant lack of female samples compared with samples from individuals aged over 5 years (1,578 of 4,048 samples under 5 years with age and sex information were female compared with 52,884 of the remaining 102,273 samples; p-value <0.001, 2-sample proportion test). These samples were especially affected by missing sex and ethnicity information on the donors compared to other age ranges (p-value <0.001, 2-sample proportion test).
One interesting significant observation was that female samples are less likely to have missing ethnicity information (30,104 of 54,462 female samples had missing ethnicity information, compared with 30,502 of 51,859 male samples; p-value <0.001, 2-sample proportion test). In a similar vein, European samples are more likely to having missing sex information than African samples (4,857 of 35,777 European samples had missing sex information, compared with 117 of 2,180 African samples; p-value <0.001, 2-sample proportion test) and than Asian samples (450 of 3,655 Asian samples missed sex information), albeit less significantly. We hypothesise that this could be due to studies focussing on groups that have faced longstanding inequities, e.g. females and underrepresented ethnicities, are more likely to also report other demographics as well.
As we mentioned when we published the Diversity Analysis Tool, our code does not offer a complete solution: more work must be done to properly understand demographic data and to subsequently adjust analyses accordingly to try to prevent perpetuating any inherent biases and furthering health inequities. All patients should benefit from drug discovery innovations, not just a subset. It is imperative that all researchers, regardless of where they sit in the drug discovery process, push to improve the diversity in data to achieve this.
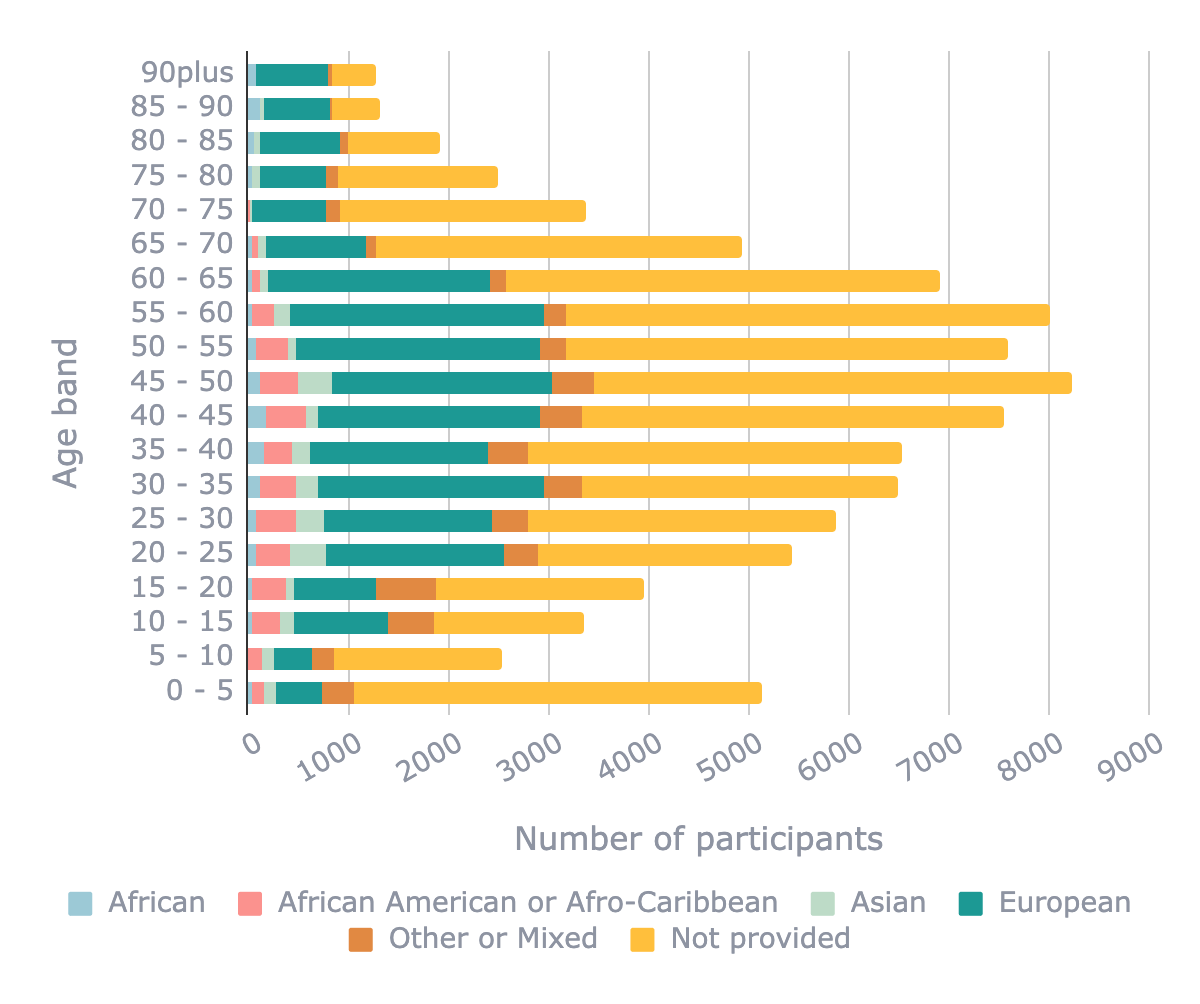
Distribution of age ranges in publicly available transcriptomic datasets coloured by ethnicity
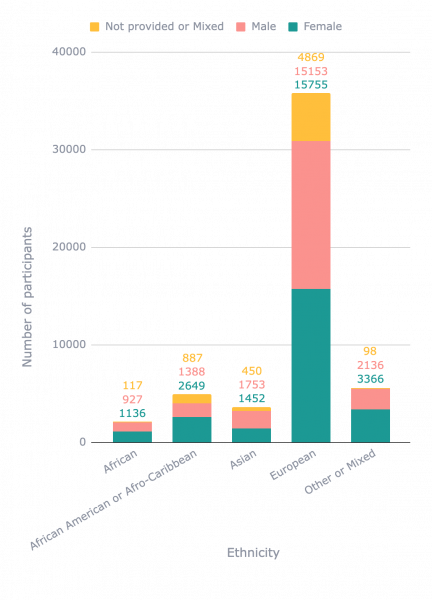
Distribution of samples of different ethnicities in publicly available transcriptomic datasets coloured by sex
While awareness of the lack of diversity in data is growing, it is still critical that researchers demonstrate the true scale of the problem and offer solutions to remedy damaging data gaps. Societal context is needed to understand bias embedded in data but it is important to record demographic factors; to look at the diversity within data being used; and to adapt analyses to avoid spreading and amplifying this bias in data-driven ML solutions. Better innovation will come from understanding the nuances and the differences age, ethnicity, sex and socio economic background can have.
Whether you are part of a research group, a pharmaceutical company, a data provider, or contribute to pharmaceutical R&D in any other way, you can take the following steps: enquire about your data, how you collect it, what you collect and question how diverse it is in order to adapt your analyses or the interpretation of conclusions. Together, these steps will help to progress towards more inclusive health research outcomes.
Test your data:
This code base we have open-sourced is meant to be simple and provide some basic code examples that may inspire you to develop more sophisticated solutions. Find out more here
Back to blog post and videos