Our team collaborated with the Sheffield Institute for Translational Neuroscience (SITraN) to co-author a review of the use of machine learning in the diagnosis and treatment of neurodegenerative diseases.
We are working collaboratively with Sitran on our ALS research, combining their deep disease expertise, assays and data, with our cutting edge technology to make a meaningful impact in the development of treatments for ALS.
The proportion of the global population over 60 years of age is increasing at a rapid pace. Along with this trend, so is the incidence of neurodegenerative disorders, such as Alzheimer’s disease and Parkinson’s disease rising. In a new review published in Nature Reviews Neurology this month, we discuss how the application of machine learning to diagnosis and treatment can help respond to this unprecedented challenge.
An overview of machine learning models for applications in neurology
Machine learning is a form of artificial intelligence that can process multiple types of data to identify patterns and relationships. We provide an overview of the three main types of machine learning models, along with their application in diagnosis, prognosis and development of new therapies.
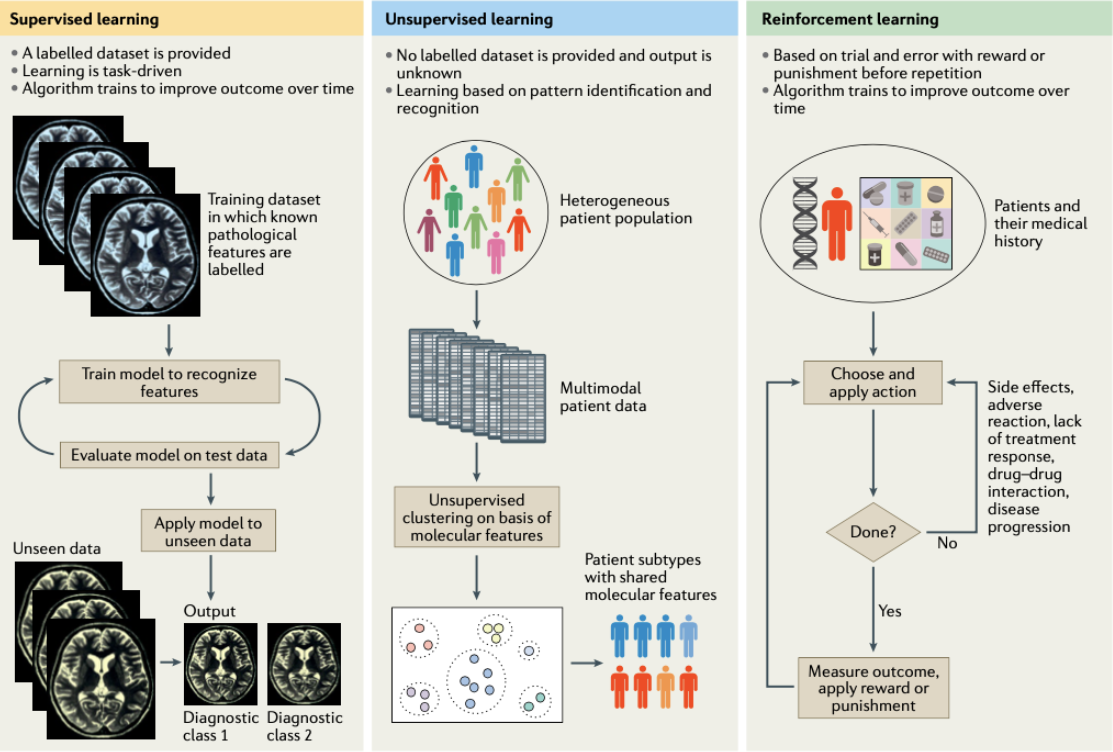
Fig 1 Categories of Machine Learning
Selecting the best machine learning model for a given problem
With rapid advances in the field of machine learning, it can be difficult for practitioners to know how to select machine learning model algorithms. Depending on the application, we provide an overview of the factors involved in this choice, such as data modality, volume, and number of features. For example, for algorithms with low data volume but with high data complexity (i.e. low sample-to-feature ratio), highly constrained models are often preferable. For higher-volume data such as medical images, deep neural networks, and in particular convolutional neural networks, tend to yield best performance. With any model choice, a skilled machine learning scientist or engineer will watch for caveats that could impact model performance, such as sub-optimal data quality or representation, and overfitting or data leakage in supervised learning. Subject matter experts can often help address these issues, by closely examining the input data, the algorithm’s predictions and helping to tune the objective function. Since all models are associated with caveats, especially in discovery applications, in practice it is often recommended to merge multiple approaches together and use a series of post-processing steps to ensure validity.
Therapy development
Due to a high failure rate of clinical trials for neurodegenerative diseases, many large pharmaceutical companies have withdrawn investment for research and development in this area. However, effective treatments are still sorely lacking. We discuss how machine learning promises to revitalize the field, in particular with novel approaches to target identification and patient stratification.
Future opportunities
New technologies are enabling the rapid generation of patient data, including medical images, wearable data, and genetic profiles. Making sense of these multi-modal and high-dimensional data sets requires careful identification and development of machine learning methods. Multi-disciplinary teams of technology and biomedical experts are necessary to bring about the transformation needed in the field.
Publication
Applications of machine learning to diagnosis and treatment of neurodegenerative diseases
Back to blog post and videos