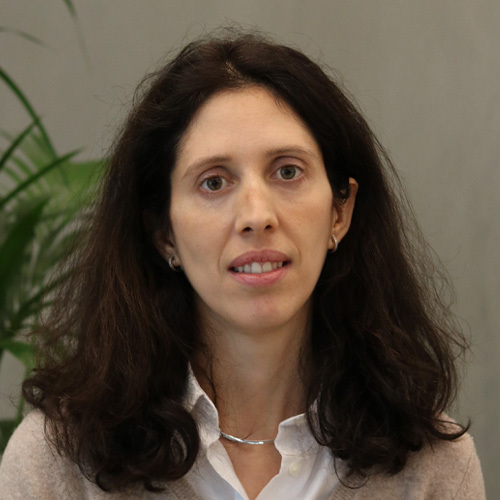
This blog discusses ‘omics data derived from single cells, and the challenges and opportunities single cell data presents in machine learning applications for drug discovery.
The importance of quality data
At BenevolentAI, data is integral to everything we do and forms the foundations for our Knowledge Graph, which drives the Benevolent Platform™, our AI-enabled drug discovery engine that allows scientists to formulate new hypotheses and rapidly discover high-quality drug targets based on a better understanding of disease. However, simply having a lot of data isn’t necessarily an advantage, as AI and machine learning tools are only effective if they operate on data that is also of sufficient quality. There is a great deal of publicly available data but much of it is not ready to incorporate into machine learning applications, and requires either substantial curation and processing by our teams, or collaborations with data providers who can generate quality curated biomedical data for us, to be able to integrate it into our Platform.
Our Knowledge Graph draws on data derived from more than 85 sources, such as different types of ‘omics data, the scientific literature, in-house experimental data and clinical trial data. Importantly, we are not constrained by the data we have already ingested into our graph and can add new data sets as well as different types of data as they become available, further enriching our data foundations.
Single cell data
One specific type of data is that derived from analyses of single cells. Although different types of ‘omics data (genomics, transcriptomics, proteomics, etc.) have been used by biomedical scientists for years, biologists are increasingly generating and analysing ‘omics data from single cells as opposed to in a mixture of many cells in bulk. Especially when conducted on human tissue samples, which include many different cell types, single cell analyses can provide very specific information on what is happening in an individual cell type of interest.
This difference is often explained using the analogy of a smoothie versus a fruit salad. If you have a smoothie containing six different types of fruit, for example, you can probably identify only some of those fruits by tasting the smoothie, but if you had all of those fruits mixed in a bowl instead, each individual fruit can be more easily identified.
Benefits of single cell data
So why are single cell data important in biomedical research and what are the benefits over batch analysis of cells?
Continuing with the fruit analogy above, in single cell transcriptomic analyses (single cell RNA sequencing, commonly called scRNA-seq, to examine gene expression), for example, you can have a view of the genes expressed in each individual cell in your sample: not only would this let you clearly see the differences between two discrete cell types (i.e. between a blueberry and a strawberry), it would also let you see differences within cells in the same population (i.e. different characteristics amongst all of the blueberries in the bowl).
Both of these aspects can be vital in drug development efforts. Considering the differences in expression of a gene of interest between two cell types in a tissue as well as the levels of expression across different individual cells of the same type is important if you are trying to target that gene for therapeutic purposes. Understanding the cell types in which potential drug targets are expressed with more precision can help us identify better drug targets for a disease as well as help prioritise targets identified by our Platform for further validation.
As an example, consider pancreatic cancer. Your drug target of interest may be expressed in the pancreas as a whole based on bulk gene expression data, but scRNA-seq data can tell you if it is expressed in the cancer cells themselves as opposed to other cell types within the pancreas, such as normal pancreatic cells, immune cells or fibroblasts. If the gene is not expressed in the cancer cells, targeting it may have no therapeutic benefit. Differences across the pancreatic cancer cells might also be important: is your target of interest expressed in all of the cancer cells or only a small percentage of them? If only a small percentage of cancer cells express the gene, there may be limited therapeutic benefit of targeting it with a drug.
Single cell transcriptomics data can also give us deeper insights into the cell types and interactions between cells that might be causal drivers or involved in the progression of different complex diseases, such as ulcerative colitis and non-alcoholic steatohepatitis (NASH), and can be used to evaluate the cellular context of different potential disease mechanisms (Figure 1). Furthermore, this type of data can tell you if the gene that you’d like to target is expressed in cells where inhibiting it might lead to safety concerns.
Integrating all of this information in our Platform provides drug discovery scientists with more insights into the best drug targets for a given disease.
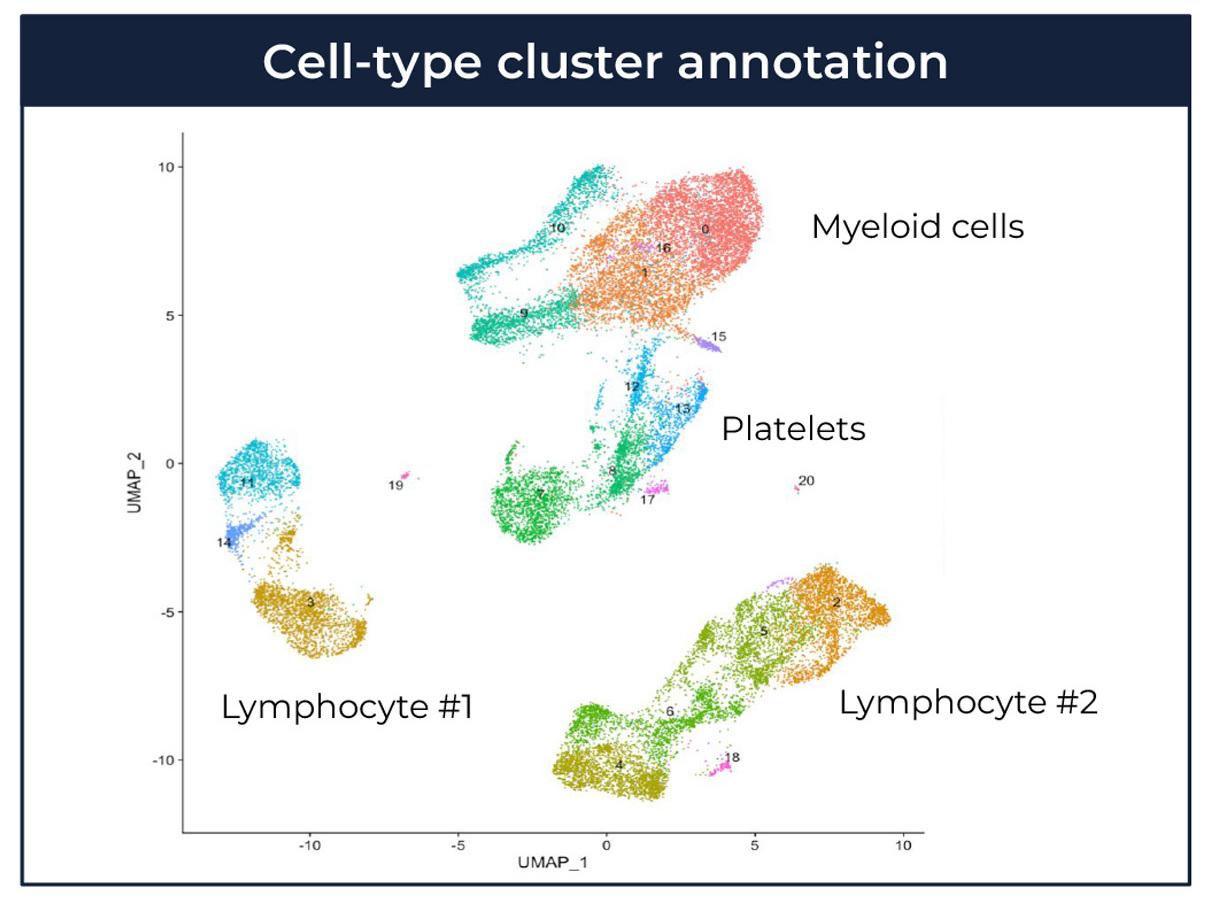
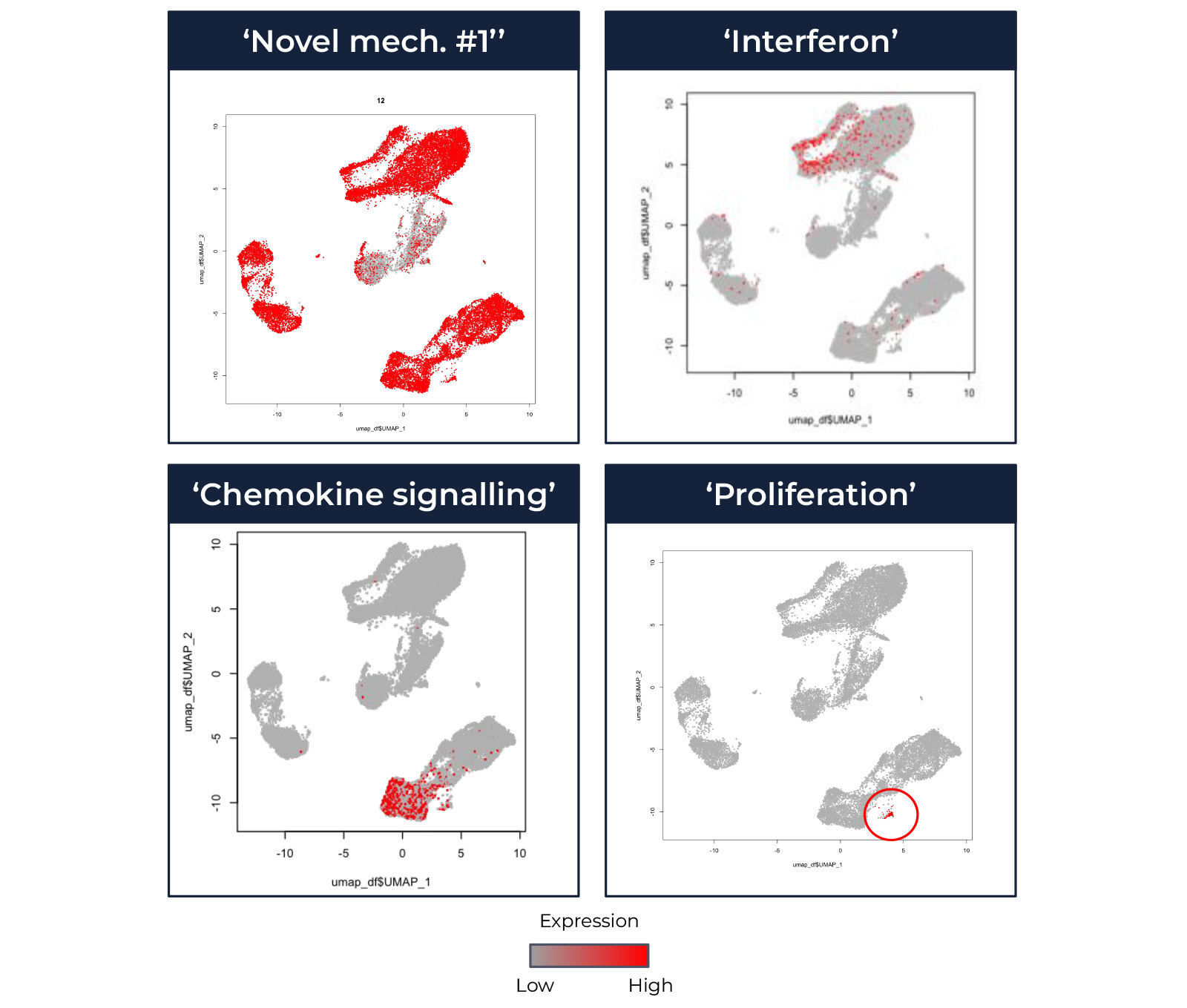
Figure 1: The cellular context of disease-associated mechanisms can aid therapeutic target identification and validation assay development. This figure shows a reanalysis of published data (Mistry et al. Proc Natl Acad Sci U S A 2019; available from https://www.ncbi.nlm.nih.gov/geo/query/acc.cgi?acc=GSE142016).Top: UMAP coordinates for scRNAseq of PBMCs from 3 patients with systemic lupus erythematosus (SLE). Each dot represents an individual cell, coloured based on cluster membership. Clusters are annotated to cell-type; this is an example of high level cell-type annotation.Bottom: UMAP coordinates for scRNAseq of PBMCs from 3 patients with SLE. Different boxes represent different mechanisms (latents), as labelled. Each dot represents an individual cell, coloured red if the top ranked genes are enriched for the latent mechanism, or grey if not.
Integrating single cell data into our Platform
The benefits of single cell data for drug discovery and the increase in accessibility of scRNA-seq technologies have led to data sets becoming more widely available. However, these data sets can be challenging to use, especially in machine learning applications, making it difficult to fully exploit these valuable sources of data.
Leveraging available single cell data sets requires finding, downloading and curating the data, which is highly laborious and time consuming. Moreover many scRNA-seq data sets lack metadata that is key for AI applications, as it provides more information on the context of the sample and allows integration of the single cell data with the other data in our graph — all of which can generate better insights for drug discovery.
We’ve been working to solve these challenges in a number of ways. For example, we recently developed a method, called Contrastive Mixture of Posteriors (CoMP), and among several potential applications of this technique, one benefit is that it helps remove the batch effects in scRNA-seq data sets and improve their integration.
We are also strong proponents of the FAIR data principles — Findability, Accessibility, Interoperability and Reusability. These principles were established with the intention of improving the infrastructure that supports data reuse, especially in machine learning applications. Our systems and data pipelines ensure our data are FAIR, and we encourage anyone collecting large data sets (from single cells or otherwise) to adhere to these principles as well so that everyone can make the best use of available data.
Finally, we’ve recently joined Rancho Biosciences’ Single Cell Data Science pre-competitive consortium, to enable us to access a large amount of well-curated scRNA-seq data along with associated high-quality metadata. This harmonised and linked metadata is a powerful tool for data stewardship since it supports all key principles of FAIR data. Incorporating the consortium’s single cell data into our Platform and integrating this new information along with our other data sources will allow us to more readily derive insights into different diseases.
Conclusion
As biomedical researchers create more and more single cell data, it is vital that we work to ensure that these data are used to their fullest potential in drug discovery efforts. Improving the availability of high-quality curated data sets with rich metadata enables us to better integrate and combine single cell data in our Knowledge Graph with all of our data from other sources. This comprehensive data landscape will provide scientists with a more complete picture of a given disease, and following from this improve our efforts to discover high-quality drug targets for that disease.
AUTHORS
Ana Leite
Director of Bioinformatics
Ana leads the Product & Tech Precision Medicine strategy at BenevolentAI, taking a patient centric approach to understand disease endotypes, mechanisms and targets. At BenevolentAI Ana also delivered a strategy for an end to end flow of Omics data, ensuring integration with the Knowledge Graph and long-term value of data assets according to FAIR principles. Ana received her PhD in Computational and Systems Biology at MIT and completed postdoctoral training at UCL Cancer Institute.
Ben Hartley
Data Licensing Manager
Ben is responsible for data licensing at BenevolentAI, supporting the company’s existing and emerging data requirements. At BenevolentAI Ben builds and maintains relationships with existing and potential data providers. Ben has more than a decade’s experience in supplier relationship management and licensing in the life sciences sector.
Back to blog post and videos