EMNLP | SustaiNLP 2020
Authors: Harshil Shah, Julien Fauqueur
Abstract
Extracting biomedical relations from large corpora of scientific documents is a challenging natural language processing task. Existing approaches usually focus on identifying a relation either in a single sentence (mention-level)or across an entire corpus (pair-level). In both cases, recent methods have achieved strong results by learning a point estimate to represent the relation; this is then used as the input to a relation classifier. However, the relation expressed in the text between a pair of biomedical entities is often more complex than can be captured by a point estimate. To address this issue, we propose a latent variable model with an arbitrarily flexible distribution to represent the relation between an entity pair. Additionally, our model provides a unified architecture for both mention-level and pair-level relation extraction. We demonstrate that our model achieves results competitive with strong base-lines for both tasks while having fewer parameters and being significantly faster to train. We make our code publicly available.
Github
The code can be accessed on GitHub here.
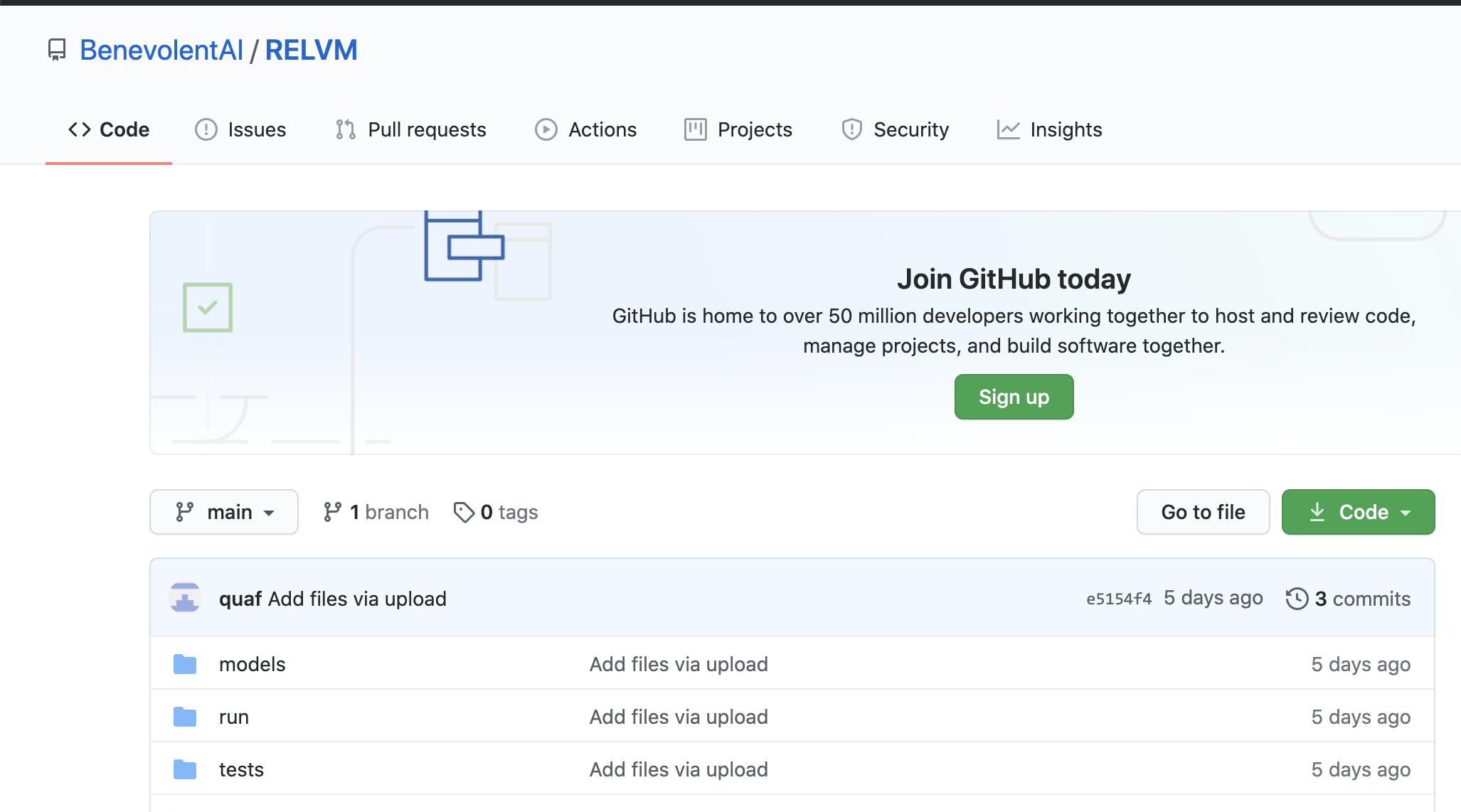
Back to publications